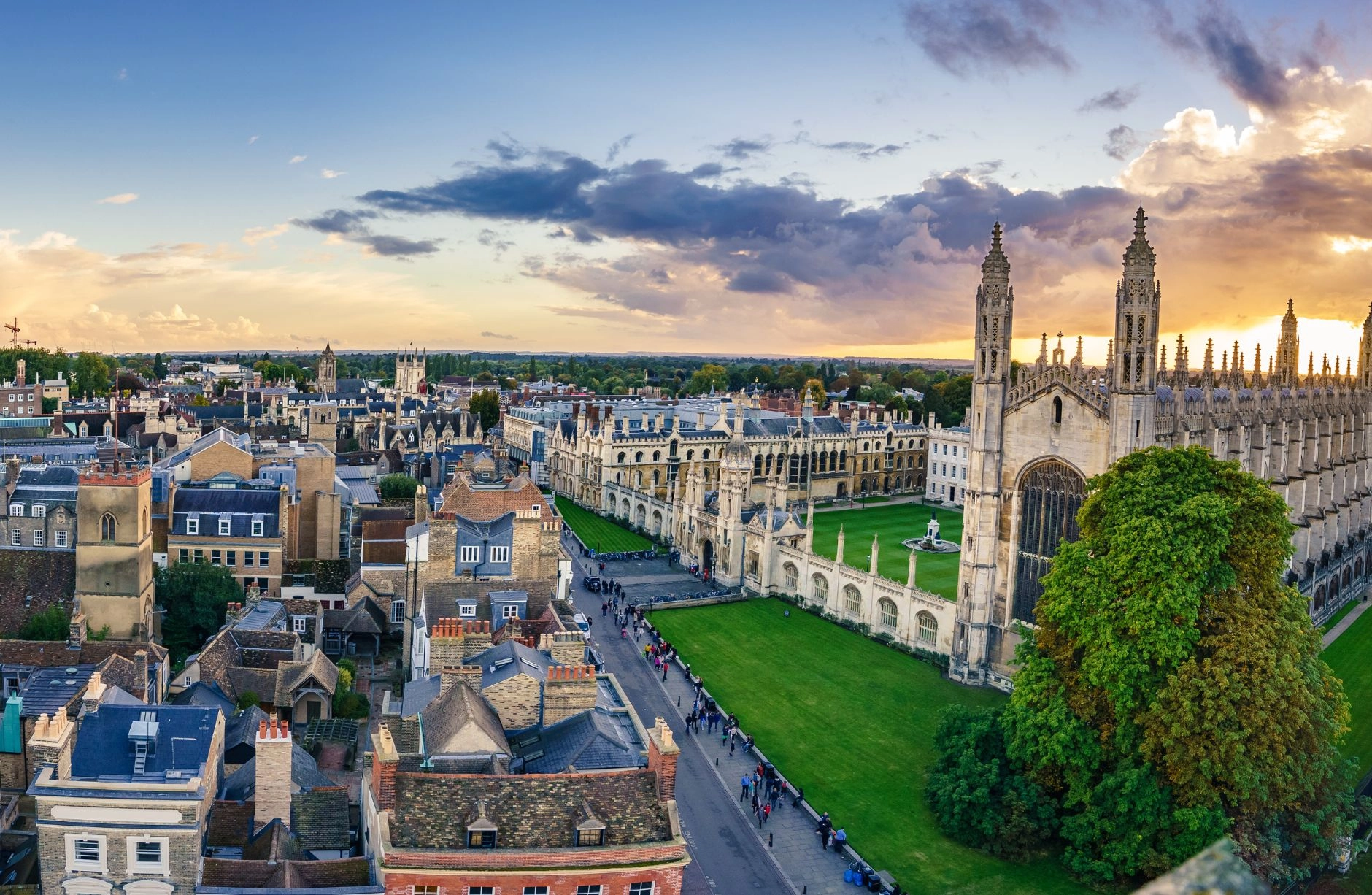
Machine Learning and Generative AI
Machine Learning (ML) and Generative AI represent two distinct but related branches of artificial intelligence, each with unique applications and implications for economists and policymakers. ML primarily focuses on prediction, causal, and classification tasks using structured data. It excels at analysing large datasets to uncover insights, make forecasts, and support decision-making processes. In economic contexts, ML is particularly useful for tasks such as assessing credit risk, detecting fraud, providing causal inference and optimizing resource allocation. Policymakers can leverage ML to evaluate policy impacts, target interventions more effectively, and improve the efficiency of public services.
Generative AI, on the other hand, is designed to create new content, whether text, images, or other forms of data. It builds on ML techniques but goes a step further by generating original outputs rather than just analyzing existing data. For economists and policymakers, Generative AI opens up new possibilities in areas such as natural language processing, scenario generation, and automated report writing. It can be used to simulate economic scenarios, generate policy briefs, or create synthetic datasets for research purposes. Large Language Models (LLMs) like GPT-4 are a prime example of Generative AI, capable of engaging in complex dialogues, answering questions, and even assisting in code writing or data analysis tasks.
While Machine Learning models typically require structured data and specific training for each task, Generative AI models like LLMs can work with unstructured data and adapt to a wide range of tasks with minimal additional training, demonstrated in the case of few-shot learning. This flexibility makes Generative AI particularly powerful for dealing with the complex, multifaceted challenges often faced in economics and policy-making. However, it's important to note that Generative AI also brings new challenges, such as the potential for generating misinformation or biased content, which requires careful consideration in policy and research contexts.
As these technologies continue to evolve, economists and policymakers will need to understand the strengths, limitations, and ethical implications of both Machine Learning and Generative AI to harness their full potential while mitigating risks.
Additionally, several global factors are complicating the outlook for emerging markets. High oil prices, a slow recovery in China, and geopolitical instability in the Middle East have further strained these economies. While the Bloomberg Emerging Markets Capital Flow Proxy Index—tracking capital flows into and out of EMs—has not yet raised major concerns, the Citi Emerging Markets Macro Risk Index has shown an increase in investor perceptions of risk, which could further amplify capital outflows (Bloomberg, 2024). Historically, a higher perception of macroeconomic risk correlates strongly with outflows, suggesting that EMs may face increasing challenges if their economic conditions continue to deteriorate.
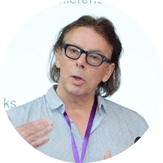
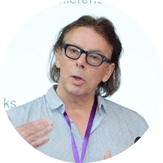
Dr Melvyn Weeks, University of Cambridge
Dr Melvyn Weeks is a senior lecturer and fellow of Clare College, Cambridge University. Dr Weeks is an assistant editor of the Journal of Applied Econometrics, as well as an associate at Cambridge Econometrics. His work has been published in The Economic Journal, Journal of the American Statistical Association, Journal of Applied Econometrics, European Economic Review, Computational & Economics.
- Bank for International Settlements. (2024). Debt in emerging markets reaches $30 trillion. Retrieved from BIS Website.
- Bloomberg. (2024). Emerging Markets Capital Flow Proxy Index: Tracking financial flows. Retrieved from Bloomberg Website.
- Financial Stability Board. (2024). Emerging markets' external debt denominated in U.S. dollars. Retrieved from FSB Website.
- International Monetary Fund. (2024). Spillover effects of U.S. monetary policy on emerging markets. Retrieved from IMF Website.
- Trading Economics. (2024). United States Dollar – Federal Reserve Interest Rate Hikes. Retrieved from Trading Economics Website.
Postagens relacionadas
-
Cambridge 2025
-
Stata 19 training
Why Timberlake is the ideal Partner to deliver Structured Hands-on Training with Stata 19
-
AI: The Future of Labour Markets
-
Farewell GPT-4.0: Which is the best ChatGPT Model for Econometrics & Data Science Programming?
-
The Green Economy: Can Sustainable Investments Drive Growth?
-
The Interest Rate Rollercoaster: How High Will They Go?
Privacy Overview
Este site utiliza cookies para melhorar a sua experiência enquanto navega no site. Dentre estes cookies, os que são classificados como necessários são armazenados no seu navegador, pois são essenciais para o funcionamento das funcionalidades básicas do site. Também utilizamos cookies de terceiros que nos ajudam a analisar e compreender como utiliza este site. Estes cookies só serão armazenados no seu navegador com o seu consentimento. Também tem a opção de recusar estes cookies. No entanto, a recusa de alguns cookies pode afetar a sua experiência de navegação.
Essential
Name | Description | Lifetime |
---|---|---|
ADD_TO_CART | (Adobe Commerce only) Used by Google Tag Manager | 1 Year |
GUEST-VIEW | Stores the Order ID that guest shoppers use to retrieve their order status. Guest orders view. Used in Orders and Returns widgets | 1 Year |
LOGIN_REDIRECT | Preserves the destination page that was loading before the customer was directed to log in | 1 Year |
MAGE-BANNERS-CACHE-STORAGE | (Adobe Commerce only) Stores banner content locally to improve performance | 1 Year |
MAGE-MESSAGES | Tracks error messages and other notifications that are shown to the user | 1 Year |
MAGE-TRANSLATION-STORAGE | Stores translated content when requested by the shopper | 1 Year |
MAGE-TRANSLATION-FILE-VERSION | Tracks the version of translations in local storage | 1 Year |
PRODUCT_DATA_STORAGE | Stores configuration for product data related to Recently Viewed/Compared Products | 1 Year |
RECENTLY_COMPARED_PRODUCT | Stores product IDs of recently compared products | 1 Year |
RECENTLY_COMPARED_PRODUCT_PREVIOUS | Stores product IDs of previously compared products for easy navigation | 1 Year |
RECENTLY_VIEWED_PRODUCT | Stores product IDs of recently viewed products for easy navigation | 1 Year |
RECENTLY_VIEWED_PRODUCT_PREVIOUS | Stores product IDs of recently previously viewed products for easy navigation | 1 Year |
REMOVE_FROM_CART | (Adobe Commerce only) Used by Google Tag Manager | 1 Year |
STF | Records the time messages are sent by the SendFriend | 1 Year |
X-MAGENTO-VARY | Configuration setting that improves performance when using Varnish static content caching | 1 Year |
FORM_KEY | A security measure that appends a random string to all form submissions to protect the data from Cross-Site Request Forgery | 1 Year |
MAGE-CACHE-SESSID | The value of this cookie triggers the cleanup of local cache storage | 1 Year |
MAGE-CACHE-STORAGE | Local storage of visitor-specific content that enables ecommerce functions | 1 Year |
MAGE-CACHE-STORAGE-SECTION-INVALIDATION | Forces local storage of specific content sections that should be invalidated | 1 Year |
PERSISTENT_SHOPPING_CART | Stores the key (ID) of persistent cart to make it possible to restore the cart for an anonymous shopper | 1 Year |
PRIVATE_CONTENT_VERSION | Appends a random, unique number and time to pages with customer content to prevent them from being cached on the server | 1 Year |
SECTION_DATA_IDS | Stores customer-specific information related to shopper-initiated actions, such as wish list display and checkout information | 1 Year |
STORE | Tracks the specific store view/locale selected by the shopper | 1 Year |
Marketing
Name | Description | Lifetime |
---|---|---|
CUSTOMER_SEGMENT_IDS | Stores your Customer Segment ID | 1 Year |
EXTERNAL_NO_CACHE | A flag that, indicates whether caching is on or off | 1 Year |
FRONTEND | Your session ID on the server | 1 Year |
GUEST-VIEW | Allows guests to edit their orders | 1 Year |
LAST_CATEGORY | The last category you visited | 1 Year |
LAST_PRODUCT | The last product you looked at | 1 Year |
NEWMESSAGE | Indicates whether a new message has been received | 1 Year |
NO_CACHE | Indicates whether it is allowed to use cache | 1 Year |
Functionality
Name | Description | Lifetime |
---|---|---|
MG_DNT | Allows you to restrict Adobe Commerce data collection if you have custom code to manage cookie consent on your site | 1 Year |
USER_ALLOWED_SAVE_COOKIE | Used for cookie restriction mode | 1 Year |
AUTHENTICATION_FLAG | Indicates if a shopper has signed in or signed out | 1 Year |
DATASERVICES_CUSTOMER_ID | Indicates if a shopper has signed in or signed out | 1 Year |
DATASERVICES_CUSTOMER_GROUP | Indicates a customer's group. This cookie is stored as sha1 checksum of the customer's group ID | 1 Year |
DATASERVICES_CART_ID | Identifies a shopper's cart actions | 1 Year |
DATASERVICES_PRODUCT_CONTEXT | Identifies a shopper's product interactions. This cookie contains the customer's unique quote ID in the system | 1 Year |
Statistical
Name | Description | Lifetime |
---|---|---|
_ga | Used by Google Analytics | 1 Year |
_ga_* | Used by Google Analytics | 1 Year |
Validate your login
Assinar em
Criar nova conta