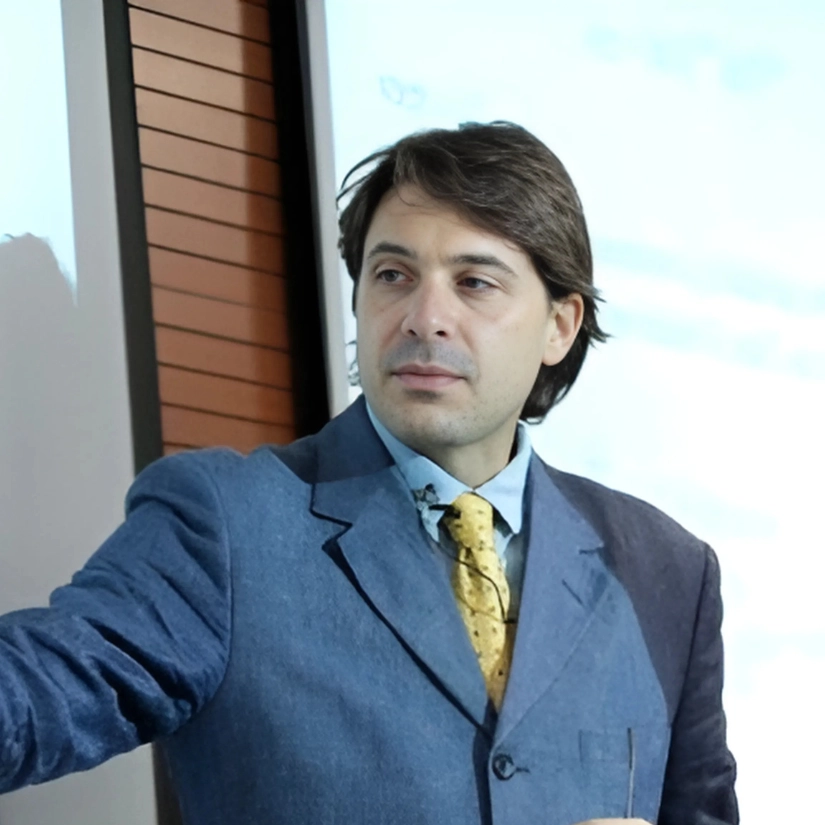
Inscreva-se aqui
- –
- 1 Day
- Online
- EViews
Overview
Time series modelling is a critical skill for understanding and forecasting macroeconomic variables, particularly when dealing with stationary processes.
This one-day online course provides a practical introduction to modelling stationary time series using EViews, with a focus on ARMA and VAR models. Participants will explore key concepts such as stationarity, unit root testing, and model estimation through hands-on exercises and real-world examples.
With applications including inflation forecasting, the course equips attendees with the tools to implement and interpret atheoretical models confidently. Ideal for those looking to enhance their forecasting skills and apply time series techniques effectively in practice.
How It Works
What You’ll Learn
-
The structure and components of ARMA (Autoregressive Moving Average) models
-
How to apply the Box-Jenkins identification procedure for time series modelling
-
Techniques for handling trends and seasonality in stationary data
-
Methods for unit root testing and achieving stationarity using differencing
-
Implementation of ARMA models for univariate forecasting in EViews
-
Evaluation of forecast accuracy and performance metrics
-
Estimation and interpretation of stationary VAR (Vector Autoregressive) models
-
Application of Granger causality tests within VAR frameworks
-
Lag length selection and its impact on VAR model quality
-
Practical forecasting using VAR models in EViews with real-world macroeconomic data
Why This Course?
This course is designed for professionals and researchers who want to deepen their expertise in modelling stationary time series data using EViews. By combining theory with practical applications, it offers a hands-on pathway to mastering ARMA and VAR models, with a special focus on macroeconomic forecasting. Whether you're aiming to enhance your analytical toolkit or improve your forecasting accuracy, this course provides the essential techniques and confidence to apply them effectively.
Course Highlights
- Comprehensive Coverage: From fundamental concepts to advanced dynamic models.
- Practical Learning: Real-world case studies and hands-on exercises with Stata.
- Expert Insights: Gain clarity on complex topics like endogeneity and serial correlation.
- Interactive Format: Live Q&A sessions to address individual questions and challenges.
Who Should Attend?
By the end of the course, participants will have a solid foundation in modelling non-stationary variables, equipping them with the skills needed to analyze complex time series data effectively.
Agenda
Non-Stationarity I - Unit Roots
Session 1: Statistical Analysis of Time Series
1.1 Definitions:
- Definition and components of Autoregressive Moving Average (ARMA) models.
- Treatment of trends and seasonality.
- Application of filters in time series analysis.
1.2 Stationarity and Non-Stationarity:
- Review of stationarity and non-stationarity in time series.
- Introduction to unit roots and their significance.
- Unit root testing in EViews.
- Series differencing as a technique for achieving stationarity.
Session 2: Univariate Forecasting I
- Forecasting with ARMA Models
- Implementation of ARMA models for univariate forecasting.
- Evaluation and measurement of forecasting accuracy.
Session 3: Atheoretical Models II: Stationary VARs
3.1 VAR Representation and Estimation:
- Introduction to Vector Autoregression (VAR) models.
- Procedures for VAR representation and estimation in EViews.
3.2 Further Testing with Multivariate Regression:
- Granger causality testing within the framework of VAR models.
- Lag selection methods to enhance VAR model performance.
Session 4: Atheoretical Models III - Stationary VARs
- Forecasting with VARs:
- Application of VAR models for forecasting in a stationary context.
- Practical considerations and techniques for VAR forecasting in EViews.
Day 2:
Session 1
- Comparing estimators for static panel models for your research question
- Testing for serial correlation
Session 2: Dynamic Panel Models
- The Arello Bond estimator and post-estimation diagnostic test
- The Blundell Bond estimator and post estimation diagnostic tests
- Case study: the determinants of bank risk-taking in European banks.
Prerequisites
Previous knowledge of regression analysis is highly desirable; some knowledge of models for stationary time series (chiefly, ARMA models) would also be beneficial.
Terms
- Student registrations: Attendees must provide proof of full time student status at the time of booking to qualify for student registration rate (valid student ID card or authorised letter of enrolment).
- Additional discounts are available for multiple registrations.
- Temporary, time limited licences for the software(s) used in the course will be provided. You are required to install the software provided prior to the start of the course.
- Payment of course fees required prior to the course start date.
- Registration closes 1-calendar day prior to the start of the course.
- 100% fee returned for cancellations made over 28-calendar days prior to start of the course.
- 50% fee returned for cancellations made 14-calendar days prior to the start of the course.
- No fee returned for cancellations made less than 14-calendar days prior to the start of the course.
The number of attendees is restricted. Please register early to guarantee your place.
Validate your login
Assinar em
Criar nova conta