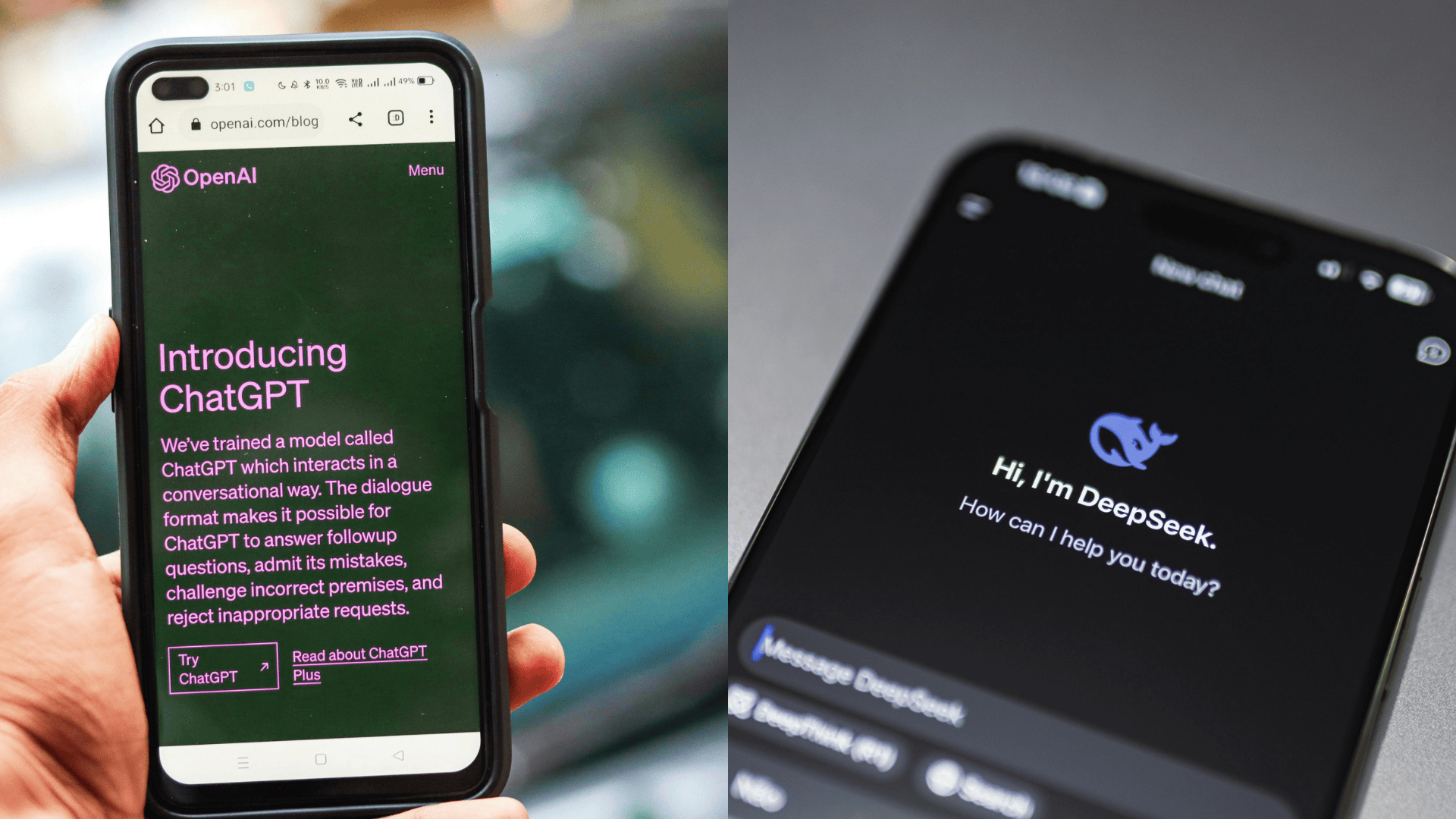
AI: The Future of Labor Markets
How Intelligent Machines Are Reshaping Work
The transformative impact of artificial intelligence (AI) on the labour market has emerged as a critical focus of both academic research and public debate. Today, AI-driven technologies are not merely supplementing traditional production processes, they are fundamentally reshaping the way industries operate, enhancing efficiency, precision, and adaptability across sectors.
From manufacturing to finance, AI is streamlining workflows and reducing human error. In manufacturing, intelligent automation has led to significant productivity gains by minimising operational mistakes and optimising supply chains (Li et al., 2020). In consumer markets, AI is revolutionising demand forecasting, personalising marketing strategies, and enabling dynamic pricing models (Abrardi et al., 2022). Within the financial sector, AI applications are redefining risk management, credit approval processes, investment strategies, and fraud detection systems using advanced data analytics (Durongkadej et al., 2024).
However, alongside these efficiency gains, AI also presents profound challenges. Labour market polarisation, the displacement of routine jobs, rising income inequality, and structural shifts in industry composition are emerging as critical risks. Far from being neutral, AI systems can also reproduce or amplify existing social biases, raising concerns about fairness and equity in increasingly automated decision-making processes.
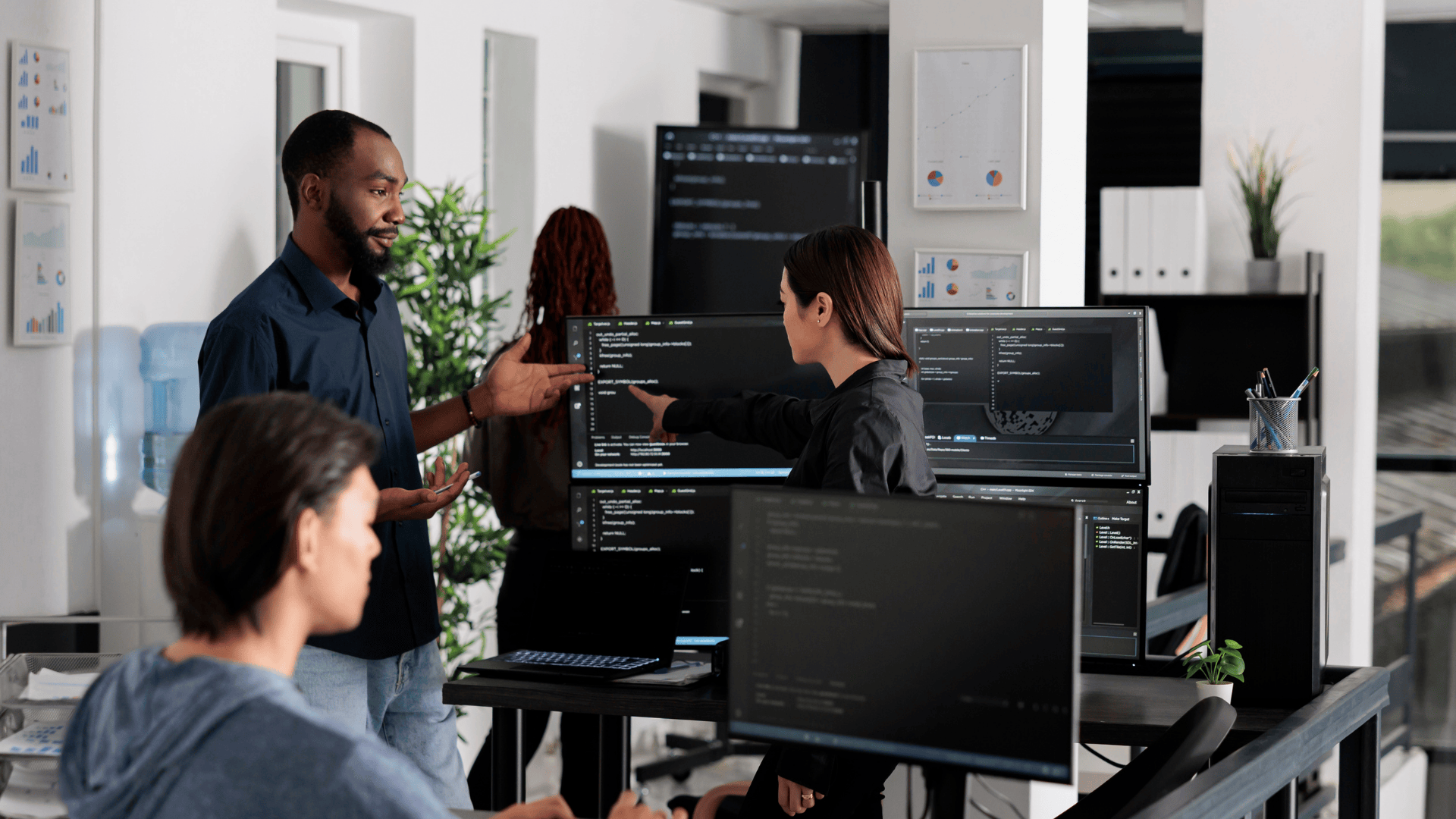
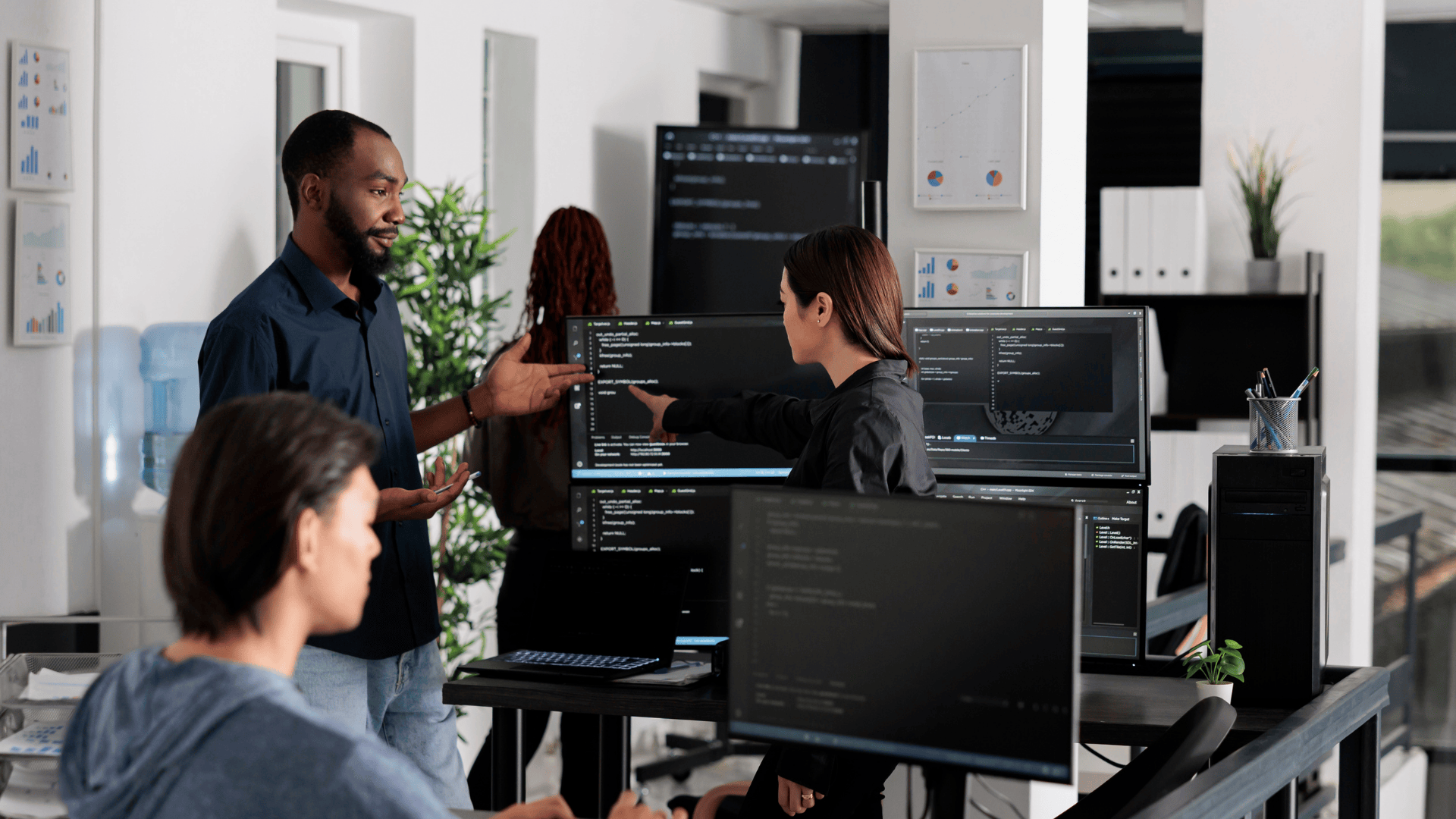
To rigorously answer these emerging questions, such as how AI influences employment structures, wage dynamics, and sectoral transitions, robust empirical analysis is essential. Advanced econometric software like Stata enables researchers to explore these complex relationships by applying panel data techniques, logit/probit models, and causal inference methods such as Difference-in-Differences (DiD). Through careful statistical modelling and data visualisation, Stata empowers analysts to move beyond speculation, providing evidence- based insights into the profound economic and social transformations driven by AI. Building expertise in tools like Stata is thus critical for anyone seeking to engage deeply with the future of labour markets and inform effective policy responses.
Regardless of whether AI is conceptualised primarily as predictive technology, automation, or an evolving form of machine intelligence, there is growing consensus that its influence on labour markets will be profound and far-reaching. Yet, predicting the precise nature of this impact remains deeply complex, and often polarising.
This blog explores the evolving relationship between AI and labour markets, highlighting both the opportunities for innovation and the critical policy challenges ahead.
What Do We Mean by Artificial Intelligence?
One of the persistent challenges in analysing the impact of artificial intelligence (AI) is the absence of a unified conceptual framework. As Agrawal et al. (2019a) point out, AI encompasses a range of evolving technologies, making it difficult to define with precision. A widely accepted view frames AI primarily as a predictive technology, driven by advances in machine learning (ML), a branch of computational statistics that enables systems to discover complex patterns within large datasets (Taddy, 2019). This allows machines to perform tasks once thought to require human intelligence, such as language processing, medical diagnosis, and even autonomous driving.
Because of its broad applications, AI defies a single, fixed definition. However, a practical and policy-relevant description is provided by the Organisation for Economic Co-operation and Development (OECD), which defines AI systems as:
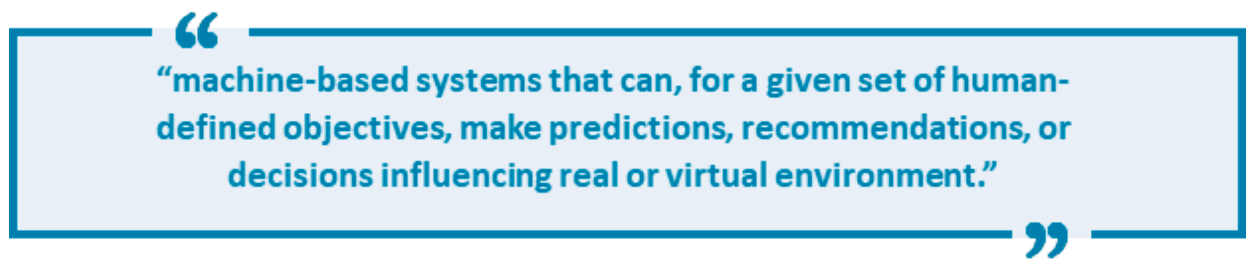
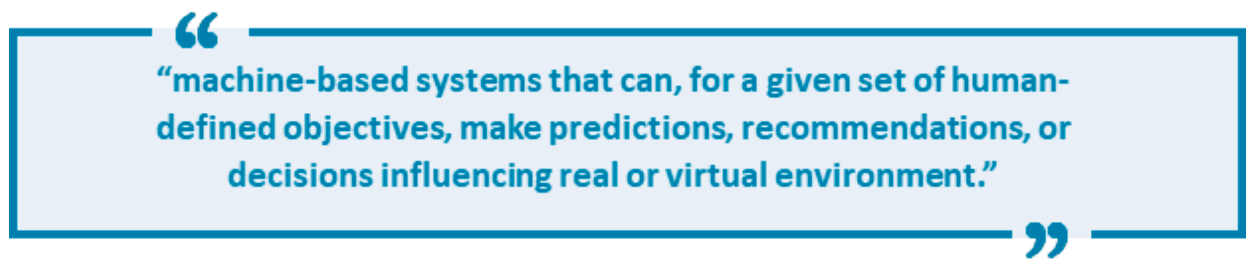
Research on AI’s economic impact is expanding rapidly, with significant attention given to its effects on employment, skill demand, wage inequality, and labour market structures. Studies by Acemoglu et al. (2022) suggest that AI tends to displace routine, repetitive tasks, contributing to a decline in low-skill jobs and the erosion of certain occupations (Zhang et al., 2024). At the same time, AI adoption is driving increased demand for high-skilled workers, particularly in data science, technology development, and AI system management, who must possess not only technical expertise but also advanced problem-solving abilities.
Beyond the labour market, AI’s repercussions are also reshaping consumer behaviour, market competition, and broader socio-economic dynamics. Many scholars warn that as AI automates more tasks, inequality and structural unemployment are likely to become central political concerns (Agrawal et al., 2019). Addressing these challenges will require proactive policies focused on wealth redistribution, reskilling programmes, and lifelong learning initiatives to prepare workers for new types of employment (Korinek & Stiglitz, 2019).
Despite mounting concerns, it remains difficult to isolate AI’s specific impact from broader trends in automation and digital transformation, given the limitations of existing empirical data. Distinguishing the effects of AI and machine learning from those of industrial robots and traditional automation technologies continues to pose a significant challenge for researchers.
AI and Job displacement
The impact of AI on employment remains a highly contested issue. While some scholars argue that AI will displace workers by automating repetitive tasks and eliminating certain occupations, others highlight its potential to spur job creation through productivity gains and the emergence of new tasks.
Evidence increasingly points toward a strong substitution effect in many sectors. Studies show that the adoption of robots and AI-driven technologies in advanced economies, including the United States, the United Kingdom, and Europe, has led to declines in labour demand, particularly in manufacturing (Chen et al., 2022). Similar concerns have been raised in emerging markets such as China, Bolivia, and Chile, where the deployment of intelligent automation is raising fears of technological unemployment (Wang et al., 2023).
Research suggests that AI-driven displacement is most acute in labour-intensive industries. For example, Stemmler (2023) found that industrial robots significantly reduced employment in manufacturing sectors, while paradoxically boosting jobs in resource extraction industries like mining. This points to structural unemployment risks, as displaced workers may struggle to transition across sectors.
Jobs characterized by routine, repetitive, and programmable tasks, such as manufacturing, logistics, and sales, are particularly vulnerable to automation (Acemoglu & Restrepo, 2019). In retail, Gong and Png (2024) show how automation in supermarkets reallocated tasks between cashiers and machines, increasing efficiency but reducing overall demand for human labour. Even traditionally non-automatable cognitive tasks, such as text generation, data analysis, and visual content creation, are increasingly being performed by AI systems.
There is broad consensus that the net effect of AI will be a shift in labour demand toward high- skilled workers, as low-skilled and routine roles are more easily replaced by machines. As with previous waves of technological disruption, this transition raises serious concerns about inequality and the redistribution of employment opportunities (Akerman et al., 2015).
AI and wage inequality
Wage dynamics have long been a central focus of labour market research, and the widespread adoption of AI technologies appears to be exacerbating existing wage inequalities. This section synthesizes evidence on how AI influences wage disparities across skill levels, industries, and regions.
AI-driven technological change affects the mobility of production factors across industries, with differences in the speed of adaptation and levels of automation contributing to growing wage divides. Industries that adapt quickly to AI, particularly skill-intensive sectors, tend to offer higher wage premiums, while labour-intensive industries experience downward pressure on wages (Cheng et al., 2024).
In sectors such as manufacturing, agriculture, and certain service industries, the introduction of automation and robotics has replaced traditional manual labour, leading to wage stagnation or decline. Jiang et al. (2024) find that greater automation of capital inputs has widened wage gaps between workers supported by automated machinery and those relying on more traditional capital. This trend is especially pronounced in non-state-owned manufacturing firms and technology-intensive enterprises, where automation investments have a particularly considerable influence on wage structures.
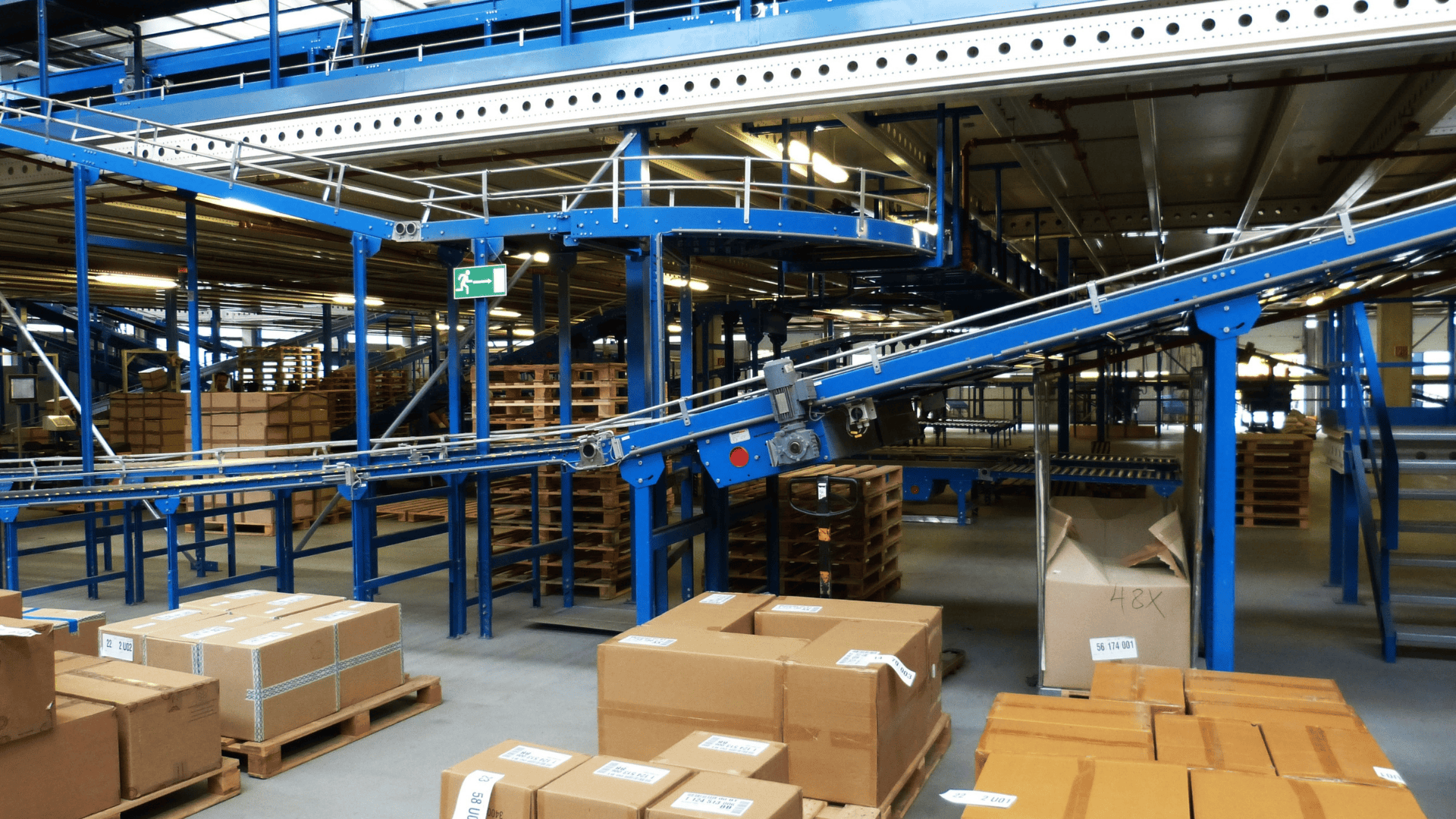
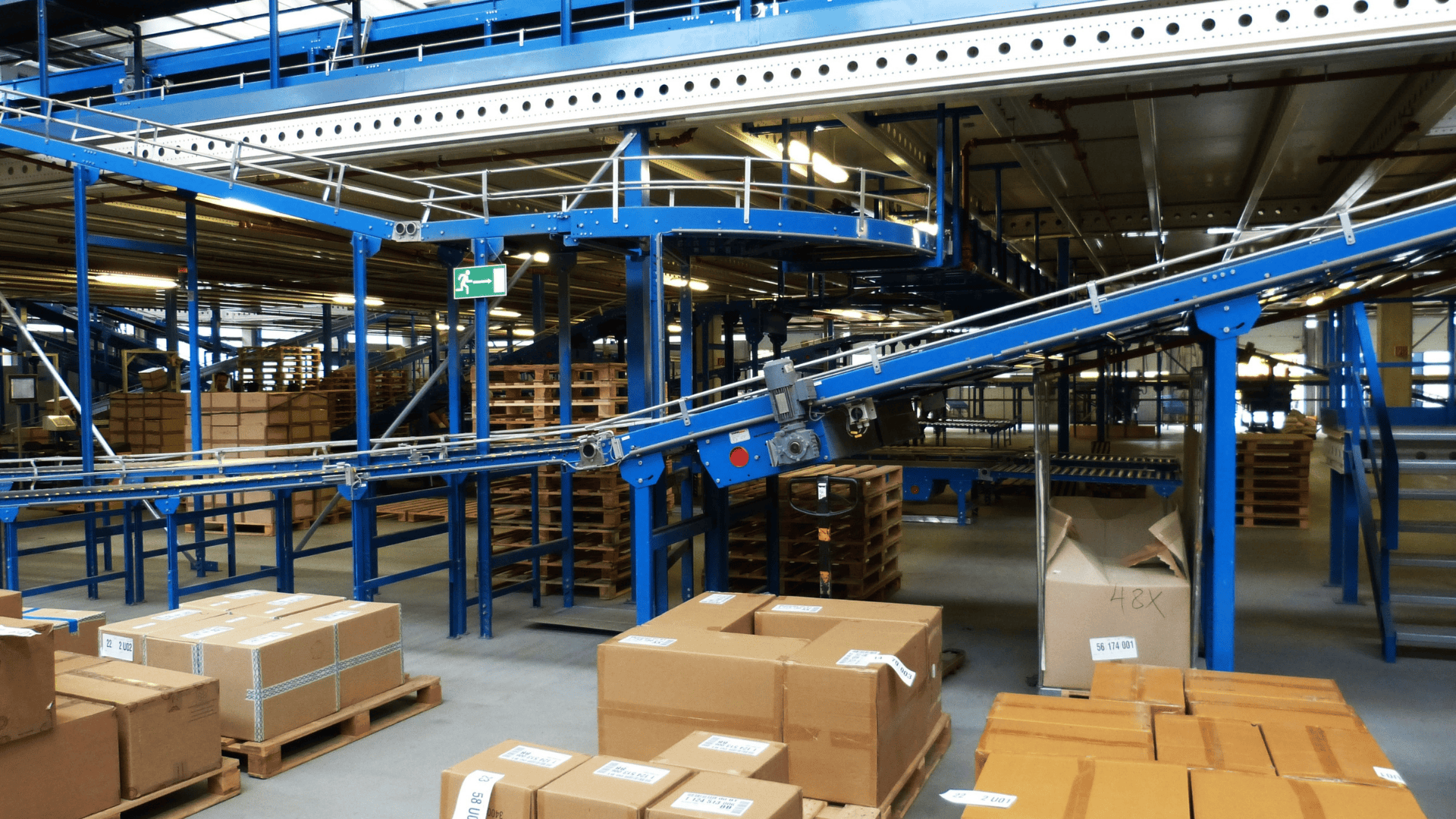
Similarly, in the service sector, AI has eroded the bargaining power of low-education frontline workers, contributing to worsening income inequality and a shrinking share of labour income within firms (Chen et al., 2024). As AI displaces basic service roles, the labour share of economic output continues to diminish, deepening wage polarization.
However, not all effects are negative. Some research highlights that AI adoption can reduce wage inequality within highly knowledge-intensive industries, such as finance and information technology. Yuan et al. (2024) suggest that in these sectors, AI-driven productivity improvements may raise overall incomes and distribute gains more evenly across the workforce. In such cases, AI functions less as a substitute for human labour and more as a complement to high-skilled workers, enhancing rather than eroding wage equity.
Overall, AI’s impact on wages is highly uneven. While it tends to depress wages in low-skill, labour-intensive industries, it can simultaneously enhance income growth and reduce inequality in high-skill, technology-driven sectors. These dual effects highlight the growing need for targeted policies to manage redistribution challenges and promote inclusive economic outcomes in the age of automation.
Conclusion: Navigating the Double-Edged Impact of AI on Labour Markets and Society
The rise of artificial intelligence (AI) has profoundly reshaped the global policy conversation on the future of work. Much of the recent debate has centred around the labour market effects of AI, particularly its role in widening wage inequalities, restructuring industries, and exacerbating risks of structural unemployment. Evidence suggests that while AI fosters productivity gains and the creation of new high-skilled jobs, it also intensifies vulnerabilities among low-skilled workers and accelerates labour market polarisation.
Yet the economic consequences of AI extend far beyond employment outcomes. As this analysis highlights, AI technologies are transforming the broader structure and functioning of markets themselves. Data-enabled learning, the core engine of AI systems, not only improves product quality and consumer access to information but also reshapes competitive dynamics across industries. Firms leverage AI not only to optimise production and services but also to redefine how they interact with customers and with each other.
However, these changes also raise new risks for consumer welfare. The widespread deployment of AI heightens concerns about algorithmic collusion, price discrimination, and exploitative business practices that may be less visible, and more difficult to regulate, than traditional anti- competitive behaviours. Thus, while AI offers considerable potential to drive innovation and 4 economic efficiency, it simultaneously demands vigilant policy oversight to safeguard equitable market outcomes.
- Looking forward, addressing the challenges posed by AI requires a multi-faceted policy approach. This includes:
- Investing in education and lifelong learning to prepare workers for the evolving demands of the AI-driven economy;
- Designing social protection systems that cushion the disruptive impacts of technological change;
- Enforcing robust competition policies to prevent anti-competitive conduct in AI-enhanced markets; and
- Ensuring transparency and accountability in algorithmic decision-making to protect consumer and worker rights.
In navigating these complex dynamics, rigorous empirical analysis is crucial for understanding the true impact of AI on labour markets, wage inequality, and broader economic structures. Sophisticated tools like Stata allow researchers and policymakers to model labour market transitions, evaluate policy interventions, and uncover hidden patterns using techniques such as panel data analysis, logit/probit regressions, and Difference-in-Differences (DiD) estimations.
For those looking to deepen their skills in these areas, the upcoming 2025 Econometrics Summer School Cambridge, organised by Timberlake Consultants, offers an outstanding opportunity. The programme, taught by leading econometricians, focuses on causal inference methods, advanced DiD techniques, and policy evaluation frameworks, all of which are directly applicable to analysing the future of labour markets shaped by AI and automation.
AI is neither an unmitigated threat nor a guaranteed solution. Its economic and social outcomes will depend heavily on how policymakers, businesses, and societies choose to manage its development and deployment. Only by balancing innovation with inclusion can we ensure that the future of work and markets shaped by AI is both dynamic and equitable.
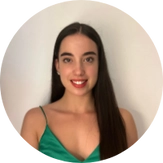
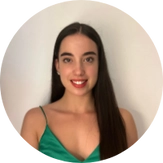
Francisca Carvalho, Lancaster University
Francisca is a third-year PhD student in Economics at Lancaster University. Her research focuses on climate risk factors and their impact on portfolio returns. She also teaches mathematics, econometrics, macroeconomics and microeconomics, to undergraduate and postgraduate students.
- Abrardi, L., Cambini, C., & Rondi, L. (2022). Artificial intelligence, firms and consumer behavior: A survey. Journal of Economic Surveys, 36(4), 969–991.
- Acemoglu, D., & Restrepo, P. (2022). Tasks, automation, and the rise in U.S. wage inequality. Econometrica, 90(5), 1973–2016. 5
- Agrawal, A., Gans, J., & Goldfarb, A. (2019a). The economics of artificial intelligence: An agenda. University of Chicago Press.
- Chen, C., Frey, C. B., & Presidente, G. (2022). Automation or globalization? The impacts of robots and Chinese imports on jobs in the United Kingdom. Journal of Economic Behavior & Organization, 204, 528–542.
- Cheng, C., Luo, J., Zhu, C., & Zhang, S. (2024). Artificial intelligence and the skill premium: A numerical analysis of theoretical models. Technological Forecasting and Social Change, 200, Article 123140.
- Durongkadej, I., Hu, W., & Wang, H. E. (2024). How artificial intelligence incidents affect banks and financial services firms? A study of five firms. Finance Research Letters, 70, Article 106279.
- Gong, J., & Png, I. P. L. (2024). Automation enables specialization: Field evidence. Management Science, 70(3), 1580–1595.
- Jiang, H., Wang, X., & Liu, C. (2024). Automated machines and the labor wage gap. Technological Forecasting and Social Change, 206, Article 123505.
- Korinek, A., & Stiglitz, J. E. (2019). Artificial intelligence and its implications for income distribution and unemployment. In A. Agrawal, J. Gans, & A. Goldfarb (Eds.), The economics of artificial intelligence: An agenda (pp. 349–390). University of Chicago Press.
- Li, X., Hui, E. C., Lang, W., Zheng, S., & Qin, X. (2020). Transition from factor-driven to innovation-driven urbanization in China: A study of manufacturing industry automation in Dongguan City. China Economic Review, 59, Article 101382.
- Stemmler, H. (2023). Automated deindustrialization: How global robotization affects emerging economies—Evidence from Brazil. World Development, 171, Article 106349.
- Taddy, M. (2019). The technological elements of artificial intelligence. In A. Agrawal, J. Gans, & A. Goldfarb (Eds.), The economics of artificial intelligence: An agenda (pp. 88–107). University of Chicago Press.
- Wang, C., Zheng, M., Bai, X., Li, Y., & Shen, W. (2023). Future of jobs in China under the impact of artificial intelligence. Finance Research Letters, 55, Article 103798.
- Yuan, Y., Sun, Y., & Chen, H. (2024). Does artificial intelligence affect firms’ inner wage gap? Applied Economics, 1–7.
- Zhang, X., Sun, M., Liu, J., & Xu, A. (2024). The nexus between industrial robots and employment in China: The effects of technology substitution and technology creation. Technological Forecasting and Social Change, 202, Article 123341.
- Zhou, H., Wang, L., Cao, Y., & Li, J. (2025). The impact of artificial intelligence on labor market: A study based on bibliometric analysis. Journal of Asian Economics, Article 101926.
Related Posts
-
-
Harnessing the Power of ChatGPT-4.0 in Econometrics and Programming: A Game-Changer for Researchers
-
The Green Economy: Can Sustainable Investments Drive Growth?
-
The Interest Rate Rollercoaster: How High Will They Go?
-
Emerging Market Challenges Amid U.S Dollar Strengths
-
Stock Market Extremes in 2024
Privacy Overview
This website uses cookies to improve your experience while you navigate through the website. Out of these cookies, the cookies that are categorized as necessary are stored on your browser as they are essential for the working of basic functionalities of the website. We also use third-party cookies that help us analyze and understand how you use this website. These cookies will be stored in your browser only with your consent. You also have the option to opt-out of these cookies. But opting out of some of these cookies may have an effect on your browsing experience.
Essential
Name | Description | Lifetime |
---|---|---|
ADD_TO_CART | (Adobe Commerce only) Used by Google Tag Manager | 1 Year |
GUEST-VIEW | Stores the Order ID that guest shoppers use to retrieve their order status. Guest orders view. Used in Orders and Returns widgets | 1 Year |
LOGIN_REDIRECT | Preserves the destination page that was loading before the customer was directed to log in | 1 Year |
MAGE-BANNERS-CACHE-STORAGE | (Adobe Commerce only) Stores banner content locally to improve performance | 1 Year |
MAGE-MESSAGES | Tracks error messages and other notifications that are shown to the user | 1 Year |
MAGE-TRANSLATION-STORAGE | Stores translated content when requested by the shopper | 1 Year |
MAGE-TRANSLATION-FILE-VERSION | Tracks the version of translations in local storage | 1 Year |
PRODUCT_DATA_STORAGE | Stores configuration for product data related to Recently Viewed/Compared Products | 1 Year |
RECENTLY_COMPARED_PRODUCT | Stores product IDs of recently compared products | 1 Year |
RECENTLY_COMPARED_PRODUCT_PREVIOUS | Stores product IDs of previously compared products for easy navigation | 1 Year |
RECENTLY_VIEWED_PRODUCT | Stores product IDs of recently viewed products for easy navigation | 1 Year |
RECENTLY_VIEWED_PRODUCT_PREVIOUS | Stores product IDs of recently previously viewed products for easy navigation | 1 Year |
REMOVE_FROM_CART | (Adobe Commerce only) Used by Google Tag Manager | 1 Year |
STF | Records the time messages are sent by the SendFriend | 1 Year |
X-MAGENTO-VARY | Configuration setting that improves performance when using Varnish static content caching | 1 Year |
FORM_KEY | A security measure that appends a random string to all form submissions to protect the data from Cross-Site Request Forgery | 1 Year |
MAGE-CACHE-SESSID | The value of this cookie triggers the cleanup of local cache storage | 1 Year |
MAGE-CACHE-STORAGE | Local storage of visitor-specific content that enables ecommerce functions | 1 Year |
MAGE-CACHE-STORAGE-SECTION-INVALIDATION | Forces local storage of specific content sections that should be invalidated | 1 Year |
PERSISTENT_SHOPPING_CART | Stores the key (ID) of persistent cart to make it possible to restore the cart for an anonymous shopper | 1 Year |
PRIVATE_CONTENT_VERSION | Appends a random, unique number and time to pages with customer content to prevent them from being cached on the server | 1 Year |
SECTION_DATA_IDS | Stores customer-specific information related to shopper-initiated actions, such as wish list display and checkout information | 1 Year |
STORE | Tracks the specific store view/locale selected by the shopper | 1 Year |
Marketing
Name | Description | Lifetime |
---|---|---|
CUSTOMER_SEGMENT_IDS | Stores your Customer Segment ID | 1 Year |
EXTERNAL_NO_CACHE | A flag that, indicates whether caching is on or off | 1 Year |
FRONTEND | Your session ID on the server | 1 Year |
GUEST-VIEW | Allows guests to edit their orders | 1 Year |
LAST_CATEGORY | The last category you visited | 1 Year |
LAST_PRODUCT | The last product you looked at | 1 Year |
NEWMESSAGE | Indicates whether a new message has been received | 1 Year |
NO_CACHE | Indicates whether it is allowed to use cache | 1 Year |
Functionality
Name | Description | Lifetime |
---|---|---|
MG_DNT | Allows you to restrict Adobe Commerce data collection if you have custom code to manage cookie consent on your site | 1 Year |
USER_ALLOWED_SAVE_COOKIE | Used for cookie restriction mode | 1 Year |
AUTHENTICATION_FLAG | Indicates if a shopper has signed in or signed out | 1 Year |
DATASERVICES_CUSTOMER_ID | Indicates if a shopper has signed in or signed out | 1 Year |
DATASERVICES_CUSTOMER_GROUP | Indicates a customer's group. This cookie is stored as sha1 checksum of the customer's group ID | 1 Year |
DATASERVICES_CART_ID | Identifies a shopper's cart actions | 1 Year |
DATASERVICES_PRODUCT_CONTEXT | Identifies a shopper's product interactions. This cookie contains the customer's unique quote ID in the system | 1 Year |
Statistical
Name | Description | Lifetime |
---|---|---|
_ga | Used by Google Analytics | 1 Year |
_ga_* | Used by Google Analytics | 1 Year |
Validate your login
Sign In
Create New Account