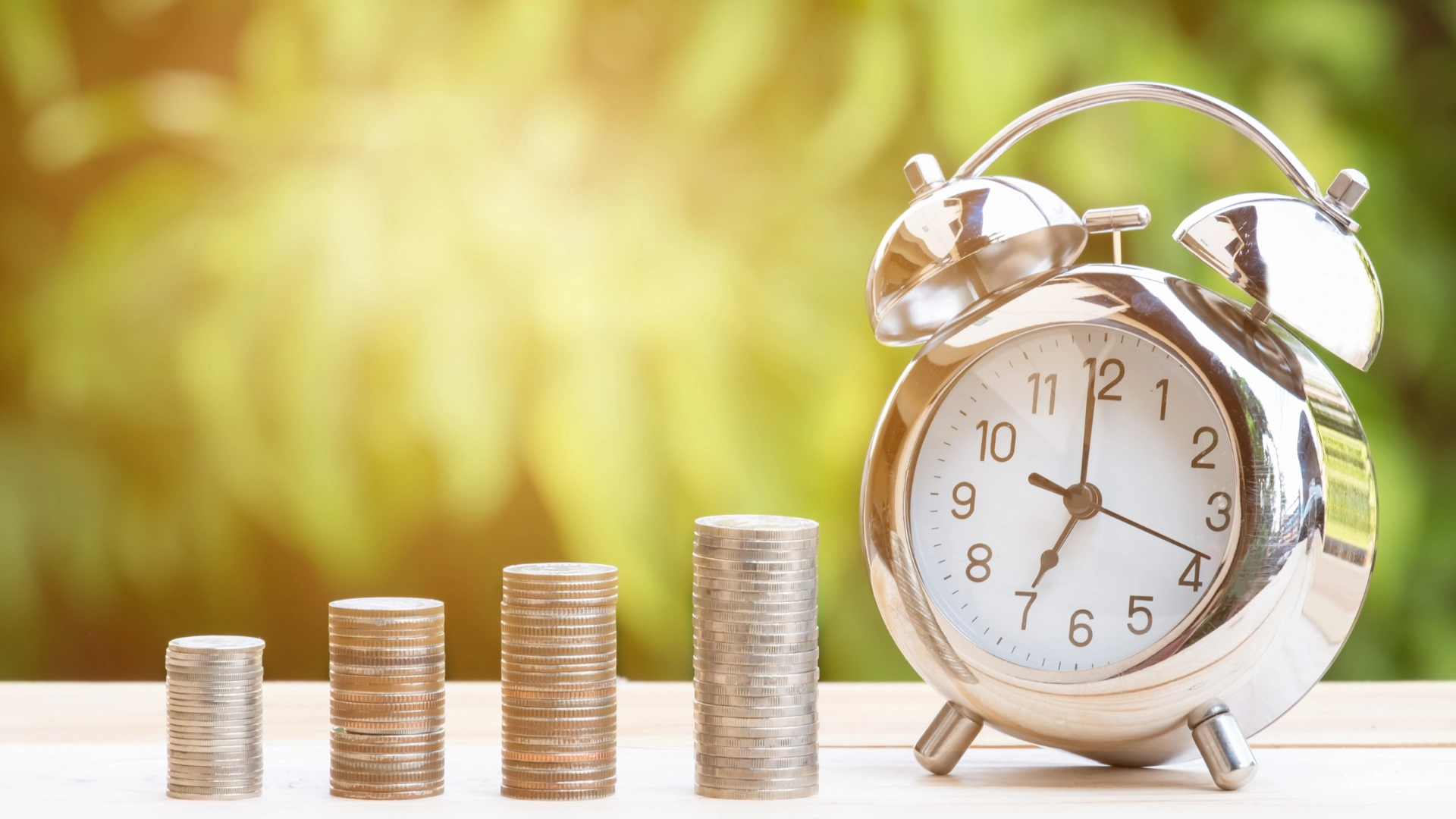
Harnessing the Power of ChatGPT-4.0 in Econometrics and Programming: A Game-Changer for Researchers
In the field of econometrics, the ability to analyze data and develop robust models is crucial for researchers and analysts. Traditionally, programming languages like Stata, Eviews, Python, and OxMetrics have been the go-to tools for econometric analysis. However, with the advancements in natural language processing (NLP) and the introduction of ChatGPT-4.0, researchers now have an incredibly powerful assistant at their disposal. In this article, we will explore the usefulness of ChatGPT-4.0 in econometrics and its implications for programming in Stata, Eviews, Python, and OxMetrics.


Understanding ChatGPT-4.0
ChatGPT-4.0 is an advanced language model developed by OpenAI. It has been trained on a vast amount of text data and can generate human-like responses to a wide range of prompts and questions. With its enhanced capabilities, ChatGPT-4.0 can understand complex queries, provide accurate information, and offer valuable insights in the domain of econometrics.
Benefits in Econometrics Programming
- Enhanced Data Manipulation: ChatGPT-4.0 can assist researchers in handling large datasets efficiently. It can help with data cleaning, transformation, merging, and subsetting, saving researchers valuable time and effort.
- Syntax Assistance: Whether it's Stata, Eviews, Python, or OxMetrics, ChatGPT-4.0 can provide real-time assistance with programming syntax. Researchers can ask questions, seek advice on correct syntax usage, and receive instant suggestions to overcome syntax-related challenges.
- Model Development and Estimation: Econometric model development and estimation can be complex tasks. ChatGPT-4.0 can aid researchers in selecting appropriate models, specifying variables, and interpreting estimation results. It can provide guidance on common issues like endogeneity, heteroscedasticity, and autocorrelation.
- Advanced Techniques and Algorithms: Econometrics often requires the application of advanced techniques and algorithms. ChatGPT-4.0 can provide insights into time series analysis, panel data analysis, instrumental variable estimation, Bayesian econometrics, machine learning approaches, and more. Researchers can learn about cutting-edge methods and implement them effectively.
- Code Optimization: Optimizing code is crucial for efficient programming. ChatGPT-4.0 can assist in improving code efficiency, suggesting alternative approaches, identifying redundant or computationally expensive code segments, and offering strategies for code optimization.
- Integration of Multiple Tools: Researchers frequently work with multiple software tools for their econometric analyses. ChatGPT-4.0 can bridge the gap by providing guidance on integrating different tools, such as using Python to preprocess data before importing it into Stata, or incorporating Python functionalities within OxMetrics workflows.
Limitations and Considerations
While ChatGPT-4.0 offers remarkable capabilities, it's important to recognize its limitations. It is not a substitute for rigorous statistical knowledge, critical thinking, or domain expertise. Researchers should exercise caution and validate the generated suggestions, as errors or incorrect interpretations can still occur. In the context of automatic code generation, it is important to systematically test the code generated by ChatGPT-4.0 and to return any error messages so that it can propose a correction. The generated code is often a starting point that saves time, but an in-depth knowledge of the programming language is required to make the changes needed to run it without error.
Conclusion
The introduction of ChatGPT-4.0 revolutionizes the way researchers approach econometrics programming. It saves a considerable amount of time and therefore increases productivity. But it is no substitute for an econometrician, and above all it does not dispense with the need to learn programming languages or econometric/statistical theory.
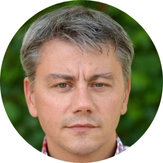
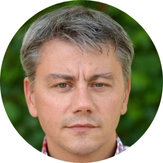
Sébastien Laurent, Aix-Marseille Université
Sébastien Laurent is a Professor at the IAE and also a member of AMSE (Greqam, France). Sébastien is an econometrician and was previously an Associate Professor in Econometrics at the Department of Quantitative Economics, Maastricht University. His research interests are financial econometrics and computational econometrics.
He is also a member of the OxMetrics development team and the main contributor of G@RCH, an OxMetrics module dedicated to the estimation and forecasting of time-series GARCH models and many of its extensions.
- Bank for International Settlements. (2024). Debt in emerging markets reaches $30 trillion. Retrieved from BIS Website.
- Bloomberg. (2024). Emerging Markets Capital Flow Proxy Index: Tracking financial flows. Retrieved from Bloomberg Website.
- Financial Stability Board. (2024). Emerging markets' external debt denominated in U.S. dollars. Retrieved from FSB Website.
- International Monetary Fund. (2024). Spillover effects of U.S. monetary policy on emerging markets. Retrieved from IMF Website.
- Trading Economics. (2024). United States Dollar – Federal Reserve Interest Rate Hikes. Retrieved from Trading Economics Website.
Related Posts
Privacy Overview
This website uses cookies to improve your experience while you navigate through the website. Out of these cookies, the cookies that are categorized as necessary are stored on your browser as they are essential for the working of basic functionalities of the website. We also use third-party cookies that help us analyze and understand how you use this website. These cookies will be stored in your browser only with your consent. You also have the option to opt-out of these cookies. But opting out of some of these cookies may have an effect on your browsing experience.
Essential
Name | Description | Lifetime |
---|---|---|
ADD_TO_CART | (Adobe Commerce only) Used by Google Tag Manager | 1 Year |
GUEST-VIEW | Stores the Order ID that guest shoppers use to retrieve their order status. Guest orders view. Used in Orders and Returns widgets | 1 Year |
LOGIN_REDIRECT | Preserves the destination page that was loading before the customer was directed to log in | 1 Year |
MAGE-BANNERS-CACHE-STORAGE | (Adobe Commerce only) Stores banner content locally to improve performance | 1 Year |
MAGE-MESSAGES | Tracks error messages and other notifications that are shown to the user | 1 Year |
MAGE-TRANSLATION-STORAGE | Stores translated content when requested by the shopper | 1 Year |
MAGE-TRANSLATION-FILE-VERSION | Tracks the version of translations in local storage | 1 Year |
PRODUCT_DATA_STORAGE | Stores configuration for product data related to Recently Viewed/Compared Products | 1 Year |
RECENTLY_COMPARED_PRODUCT | Stores product IDs of recently compared products | 1 Year |
RECENTLY_COMPARED_PRODUCT_PREVIOUS | Stores product IDs of previously compared products for easy navigation | 1 Year |
RECENTLY_VIEWED_PRODUCT | Stores product IDs of recently viewed products for easy navigation | 1 Year |
RECENTLY_VIEWED_PRODUCT_PREVIOUS | Stores product IDs of recently previously viewed products for easy navigation | 1 Year |
REMOVE_FROM_CART | (Adobe Commerce only) Used by Google Tag Manager | 1 Year |
STF | Records the time messages are sent by the SendFriend | 1 Year |
X-MAGENTO-VARY | Configuration setting that improves performance when using Varnish static content caching | 1 Year |
FORM_KEY | A security measure that appends a random string to all form submissions to protect the data from Cross-Site Request Forgery | 1 Year |
MAGE-CACHE-SESSID | The value of this cookie triggers the cleanup of local cache storage | 1 Year |
MAGE-CACHE-STORAGE | Local storage of visitor-specific content that enables ecommerce functions | 1 Year |
MAGE-CACHE-STORAGE-SECTION-INVALIDATION | Forces local storage of specific content sections that should be invalidated | 1 Year |
PERSISTENT_SHOPPING_CART | Stores the key (ID) of persistent cart to make it possible to restore the cart for an anonymous shopper | 1 Year |
PRIVATE_CONTENT_VERSION | Appends a random, unique number and time to pages with customer content to prevent them from being cached on the server | 1 Year |
SECTION_DATA_IDS | Stores customer-specific information related to shopper-initiated actions, such as wish list display and checkout information | 1 Year |
STORE | Tracks the specific store view/locale selected by the shopper | 1 Year |
Marketing
Name | Description | Lifetime |
---|---|---|
CUSTOMER_SEGMENT_IDS | Stores your Customer Segment ID | 1 Year |
EXTERNAL_NO_CACHE | A flag that, indicates whether caching is on or off | 1 Year |
FRONTEND | Your session ID on the server | 1 Year |
GUEST-VIEW | Allows guests to edit their orders | 1 Year |
LAST_CATEGORY | The last category you visited | 1 Year |
LAST_PRODUCT | The last product you looked at | 1 Year |
NEWMESSAGE | Indicates whether a new message has been received | 1 Year |
NO_CACHE | Indicates whether it is allowed to use cache | 1 Year |
Functionality
Name | Description | Lifetime |
---|---|---|
MG_DNT | Allows you to restrict Adobe Commerce data collection if you have custom code to manage cookie consent on your site | 1 Year |
USER_ALLOWED_SAVE_COOKIE | Used for cookie restriction mode | 1 Year |
AUTHENTICATION_FLAG | Indicates if a shopper has signed in or signed out | 1 Year |
DATASERVICES_CUSTOMER_ID | Indicates if a shopper has signed in or signed out | 1 Year |
DATASERVICES_CUSTOMER_GROUP | Indicates a customer's group. This cookie is stored as sha1 checksum of the customer's group ID | 1 Year |
DATASERVICES_CART_ID | Identifies a shopper's cart actions | 1 Year |
DATASERVICES_PRODUCT_CONTEXT | Identifies a shopper's product interactions. This cookie contains the customer's unique quote ID in the system | 1 Year |
Statistical
Name | Description | Lifetime |
---|---|---|
_ga | Used by Google Analytics | 1 Year |
_ga_* | Used by Google Analytics | 1 Year |
Validate your login
Sign In
Create New Account